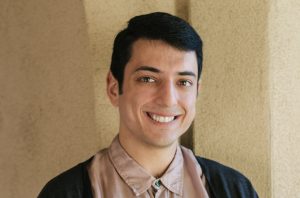
Raised in Garland, Texas, Matthew is an ardent scientist. He attended the University of Texas at Dallas (UTD) for his undergraduate degree in 2012 having received an Academic Excellence Scholarship to study Physics. In 2015, he received a NSF Research Experience for Undergraduates award and worked with Dr. Benjamin Sargent at the Rochester Institute of Technology on the study of Asymptotic Giant Branch stars. This research resulted in a poster presentation at the 227th American Astronomy Society conference in 2016. He then graduated from UTD with a B.S. in Physics with distinction from the Collegium V Honors College.
He was then accepted into the Computational Science Ph.D. program at the University of California, Irvine (UCI) joint with San Diego State University (SDSU) in 2017. Matthew currently works with Dr. Wayne Hayes on the study of spiral galaxy morphology using computer vision and optimization techniques and has presented this work at the 2019 and 2021 poster sessions of the annual ACCESS conference at SDSU. His awards include: a G-STEM fellowship from SDSU, a Division of Teaching Excellence and Innovation fellowship from UCI, and a Visiting Scholars Program award from the Universities Research Association in conjunction with Fermilab.
Research Advisor(s)
Wayne Hayes
Research Abstracts
RSpiral Galaxy Morphology:
Spiral galaxies, including our own Milky Way, comprise about 70% of galaxies observed in the local universe yet the formation of the characteristic arms and their visible structure are not fully understood beyond strict formalisms developed to describe their dynamics. Our work aims to provide objective, quantitative measures of their structure in order to provide further insight into their morphology due in part to the wide variety of spiral structures observed. To do this, we use Spiral Arc Finder and Reporter (SpArcFiRe), a computer vision algorithm which takes images of spiral galaxies and automatically extracts simple objective information about their structure and GALFIT, a parameteric fitting algorithm which fits a multi-component light model to the observations fed in to automate the process. This approach allows us the flexibility to generalize the fitting procedure by focusing on modeling features common to all spiral galaxies. Our goal with this research is to fully automate this process in order to meet the demands of existing and upcoming sky surveys by providing information in bulk on the millions of galaxies observed.
Gravitational Wave follow-up of Transient Phenomena:
Our research, in collaboration with scientists at Lawrence Berkeley National Lab, focuses on time domain astronomy with an emphasis on transient phenomena in Dark Energy Spectroscopic Instrument (DESI) observations and Gravitational Wave (GW) follow-up. For this research, we have developed an algorithm which identifies DESI observations and transient alerts in the confidence interval regions of a GW event. The algorithm is part of a larger pipeline we are developing which will ingest transients from GW observations and several types of sky surveys such as the La Silla Schmidt Southern Survey, Dark Energy Camera surveys, and Rubin Observatory LSST; provide instructions for the DESI observations based on these transients; provide a machine learning classification of the transients through an existing Convolutional Neural Network classifier; and update the SkyPortal online database. Our goal is to complete the pipeline in time for the return of the Laser Interferometer Gravitational Observatory (LIGO), set to come back online in 2022.
Click image to view research report presentation!
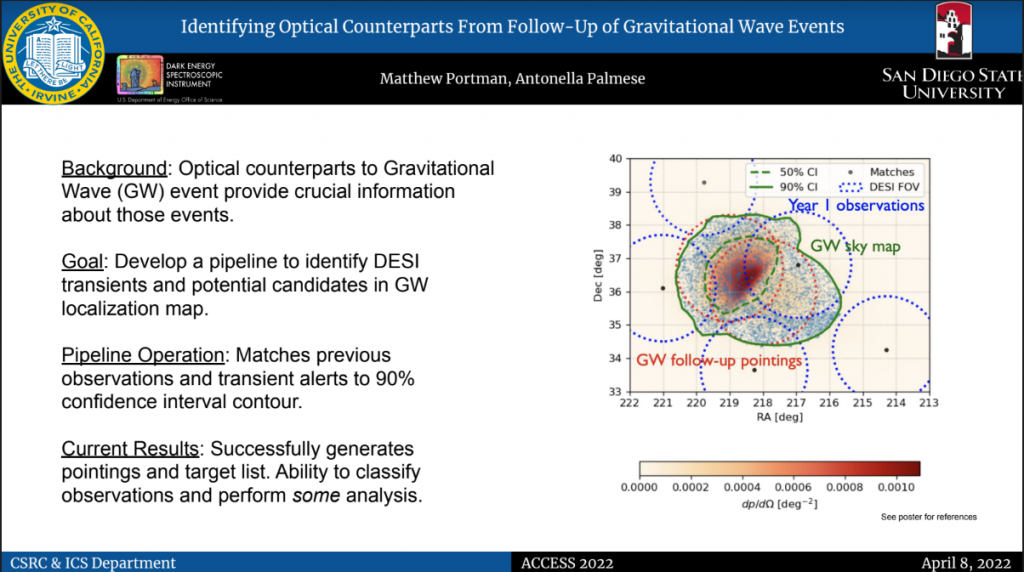