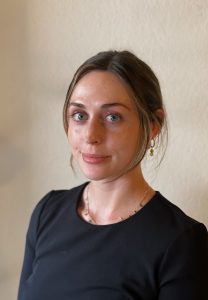
I am currently in my 3rd year of my PhD through the Computational Science Research Center at SDSU/UCI. In 2019, graduated summa cum laude from SDSU with a BS in physics and a minor in interdisciplinary studies. During my time in undergrad, I began researching neutron stars under Dr. Fridolin Weber; my undergraduate thesis explored the potential for deconfined quarks in the superdense cores of these stars. Dr. Weber is still my advisor for my PhD alongside Dr. Daniel Whiteson from UCI. I currently work on two main projects: (1) using machine learning to predict neutron star matter equation of state from simulated x-ray spectra, and (2) numerically implementing differential rotation into existing rotation codes for neutron stars.
Research Advisor(s)
Fridolin Weber and Daniel Whiteson
Research Abstract
Recently, I have been researching into the application of machine learning techniques such as K-means clustering, Support Vector A neutron star equation of state (EOS), or the relationship between pressure and energy density within the star, determines observable structural properties like mass and radius. These same structural properties can also be determined by observation of x-ray emission or gravitational waves produced by neutron stars. Despite the clear link between the theoretical depiction of the EOS and observable spectra, there is no clear mathematical relation where spectra can determine EOS, or vice versa. We propose using machine learning to infer neutron star EOS from x-ray spectra simulated to match real observations from NASA’s x-ray observatory Chandra. We devise two piecewise neural networks trained on three corresponding data sets: a density dependent nuclear EOS, mass and radius values solved for with the Tolman–Oppenheimer–Volkoff (TOV) equation, and simulated x-ray spectra augmented with uncertainties consistent with those seen in observation. Both networks aim to predict mass and radius or EOS from spectra, as well as moving in the opposite direction – predicting spectra or mass and radius from EOS. The goal of this project is to extract the most likely EOS from observed data to constrain the existing range of theoretical neutron star EOS.
Click image to view research report presentation!