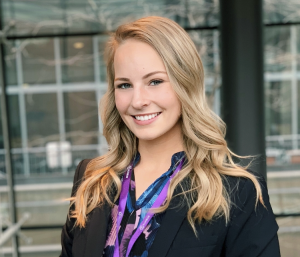
Amanda Lee is a student in the Joint Doctoral Program in Computational Science. Amanda’s research interests include using machine learning techniques and statistical analysis to investigate the genetic and environmental factors of chronic obstructive pulmonary disease (COPD). Her current work includes the development of a convolutional neural network that will identify novel biomarkers in medical images of COPD lungs. After receiving her Ph.D., Amanda’s goal is to build a career applying artificial intelligence, machine learning, and data science to problems in biomedical research in industry or at a national lab.
Research Advisor(s)
Kyle Hasenstab and Pierre Baldi
Research Abstract
Chronic obstructive pulmonary disease (COPD) is the third leading cause of disease-related death in the United States. COPD severity is primarily assessed using a patient’s risk factors for disease, severity of symptoms, and measurements of lung function. However, recent studies have shown lung structure, quantitatively measured using lung computed tomography (CT) imaging, can be used to aid the evaluation of COPD. CT images are potentially rich with structural information useful for disease characterization; however, when used to assess COPD, they are typically reduced to only two biomarkers using basic summary statistics: (1) the percentage of the lungs affected by emphysema and (2) the percentage of the lungs affected by air trapping. We hypothesize that an alternative approach that utilizes the entire image distribution of the lungs would improve COPD diagnosis, prognosis, and staging. Our primary goal is to develop state-of-the-art machine learning algorithms to investigate new CT imaging biomarkers for COPD. The interpretation of these networks will allow us to determine which CT imaging biomarkers are the most important for disease characterization. A greater understanding of which structural features within the lung are critical to lung function would enhance the possibility of personalized diagnoses and treatment plans.
Click image to view research report presentation!